Demonstrating the utility of machine learning innovations in address matching to spatial socio-economic applications
DOI:
https://doi.org/10.18335/region.v6i3.276Abstract
The last decade has heralded an unprecedented rise in the number, frequency and availability of data sources. Yet they are often incomplete, meaning data fusion is required to enhance their quality and scope. In the context of spatial analysis, address matching is critical to enhancing household socio-economic and demographic characteristics. Matching administrative, commercial, or lifestyle data sources to items such as household surveys has the potential benefits of improving data quality, enabling spatial data visualisation, and the lowering of respondent burden in household surveys. Typically when a practitioner has high quality data, unique identifiers are used to facilitate a direct linkage between household addresses. However, real-world databases are often absent of unique identifiers to enable a one-to-one match. Moreover, irregularities between the text representations of potential matches mean extensive cleaning of the data is often required as a pre-processing step. For this reason, practitioners have traditionally relied on two linkage techniques for facilitating matches between the text representations of addresses that are broadly divided into deterministic or mathematical approaches. Deterministic matching consists of constructing hand-crafted rules that classify address matches and non-matches based on specialist domain knowledge, while mathematical approaches have increasingly adopted machine learning techniques for resolving pairs of addresses to a match. In this notebook we demonstrate methods of the latter by demonstrating the utility of machine learning approaches to the address matching work flow. To achieve this, we construct a predictive model that resolves matches between two small datasets of restaurant addresses in the US. While the problem case may seem trivial, the intention of the notebook is to demonstrate an approach that is reproducible and extensible to larger data challenges. Thus, in the present notebook, we document an end-to-end pipeline that is replicable and instructive towards assisting future address matching problem cases faced by the regional scientist.
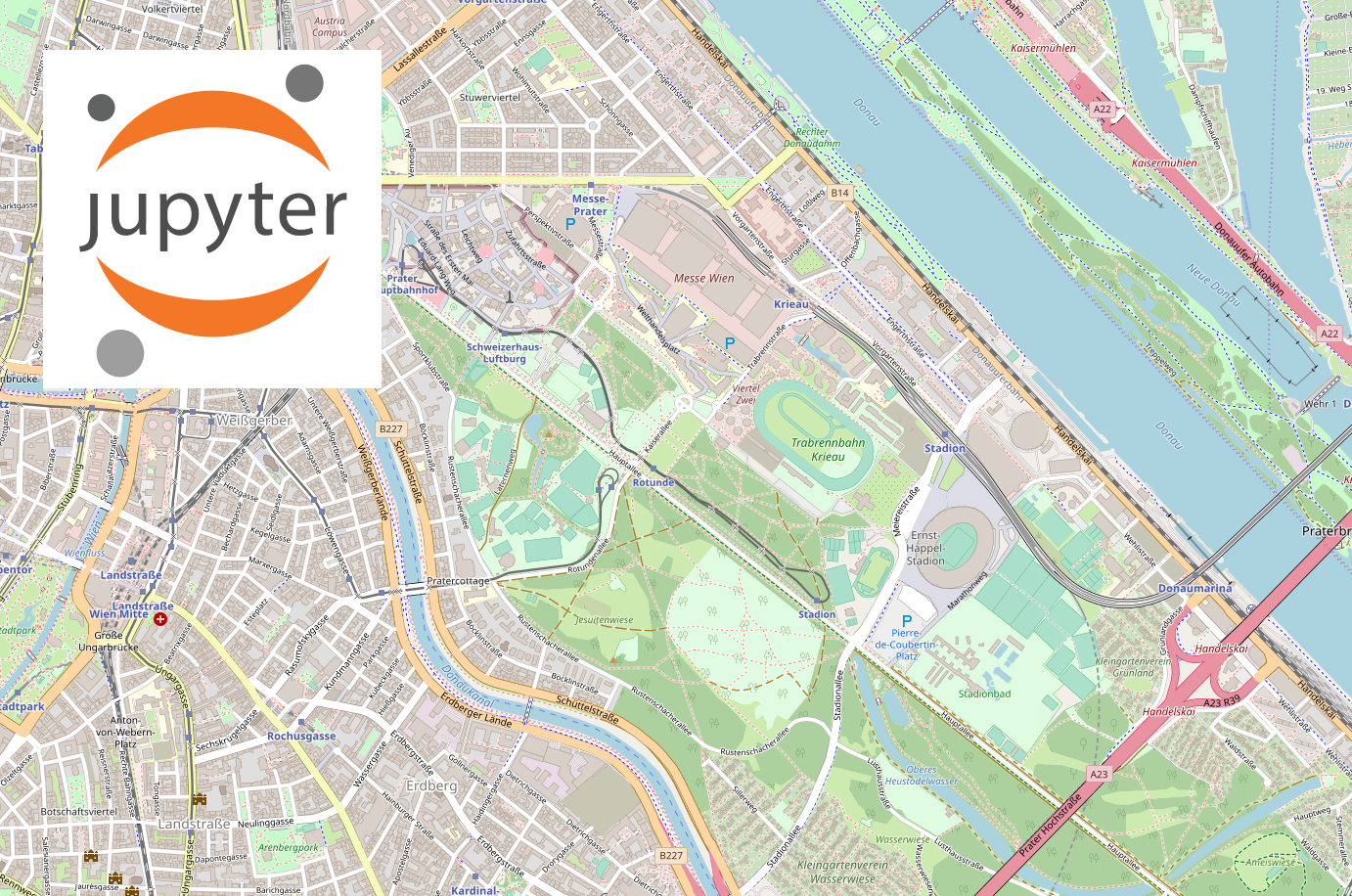
Published
Versions
- 2020-01-13 (2)
- 2020-01-13 (1)
How to Cite
Issue
Section
License
Copyright (c) 2020 Sam Comber

This work is licensed under a Creative Commons Attribution-NonCommercial 4.0 International License.
REGION is an open journal, and uses the standard Creative Commons license: Copyright We want authors to retain the maximum control over their work consistent with the first goal. For this reason, authors who publish in REGION will release their articles under the Creative Commons Attribution license. This license allows anyone to copy and distribute the article provided that appropriate attribution is given to REGION and the authors. For details of the rights authors grant users of their work, see the "human-readable summary" of the license, with a link to the full license. (Note that "you" refers to a user, not an author, in the summary.) Upon submission, the authors agree that the following three items are true: 1) The manuscript named above: a) represents valid work and neither it nor any other that I have written with substantially similar content has been published before in any form except as a preprint, b) is not concurrently submitted to another publication, and c) does not infringe anyone’s copyright. The Author(s) holds ERSA, WU, REGION, and the Editors of REGION harmless against all copyright claims. d) I have, or a coauthor has, had sufficient access to the data to verify the manuscript’s scientific integrity. 2) If asked, I will provide or fully cooperate in providing the data on which the manuscript is based so the editors or their assignees can examine it (where possible) 3) For papers with more than one author, I as the submitter have the permission of the coauthors to submit this work, and all authors agree that the corresponding author will be the main correspondent with the editorial office, and review the edited manuscript and proof. If there is only one author, I will be the corresponding author and agree to handle these responsibilities.